Investigating the Spectrum of Earthquakes Using Data Science

Bertrand Philippe Gerard ROUET-LEDUC
地震災害研究センター宇宙測地研究領域
特定助教
Introduction of three AI-related projects at DPRI:
1) The development and application of deep learning methods to automatically detect surface deformation in satellite radar interferometric data, with the goal of improving the understanding of the interactions between slow and fast earthquakes. The methods is being improved and applied to detect deformation on the North Anatolian Fault in Turkey, and on the San Andreas Fault in California (Fig. 1). These results show that slow and fast earthquakes interact, and that slow deformation can be a precursor to fast and damaging earthquakes. The method also enables for the first time to build catalogs of slow earthquakes on continental faults, therefore opening the way for a better understanding of the continuum of slip on faults.
2) The development of an earthquake early warning system based on the detection of so-called prompt elasto-gravity signals. The method enables the accurate and real time estimation of earthquake magnitude (Fig. 2), which can then also be used for tsunami early warning. Classic earthquake early warning suffers from limitations such as a priori constraints on source finiteness that lead to poor estimations for very large earthquakes (Mw 8+). On the contrary, elasto-gravity based estimations start working above Mw 8 and improve with magnitude. These systems could therefore come in complement to existing earthquake early warning systems in order to improve the accuracy for very large earthquakes.
3) As an additional project a deep learning method to detect methane leaks in multi spectral data is being developed. Methane accounts for a third of global warming and is shorter lived in the atmosphere than CO2. The method will unlock the possibility of global and daily automatic detection of methane leaks.
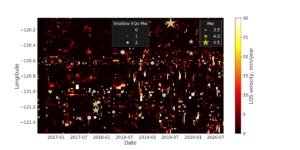
Figure 1 Detection of slow earthquakes on the San Andreas Fault using a deep learning method that extract small deformation signals from noisy InSAR (Interferometric Synthetic Aperture Radar) time series. The background color shows slip on the fault, while white dots show surface microseismicity (less than 1km deep) and the yellow stars show the deeper earthquakes (around 10kms). Systematically and automatically detecting slow earthquakes can improve the understanding of the interplay between slow and fast earthquakes.
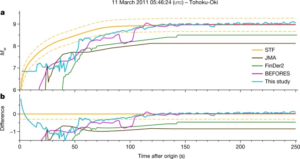
Figure 1 Detection of slow earthquakes on the San Andreas Fault using a deep learning method that extract small deformation signals from noisy InSAR (Interferometric Synthetic Aperture Radar) time series. The background color shows slip on the fault, while white dots show surface microseismicity (less than 1km deep) and the yellow stars show the deeper earthquakes (around 10kms). Systematically and automatically detecting slow earthquakes can improve the understanding of the interplay between slow and fast earthquakes.